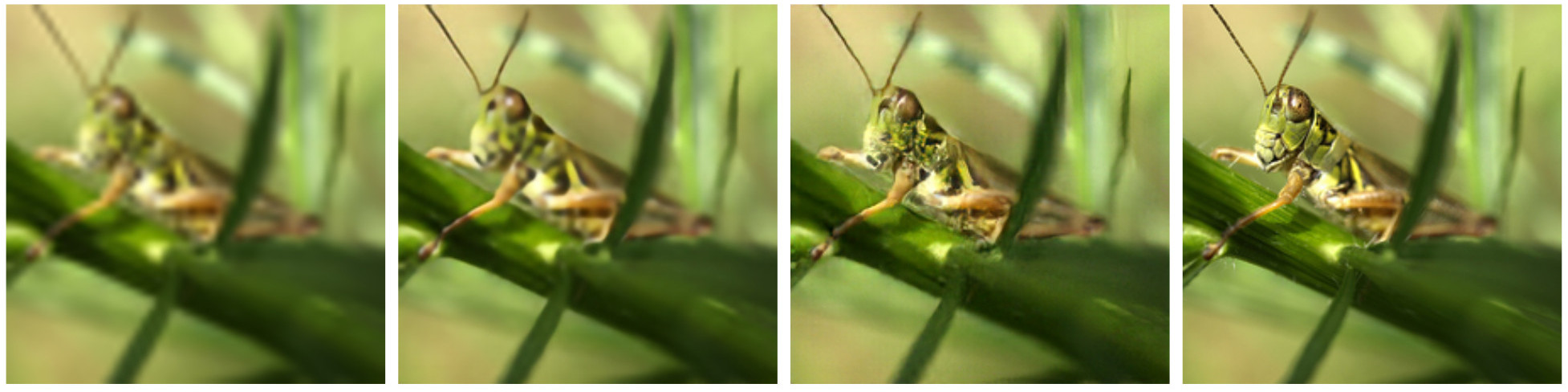
Super-resolution aims at estimating a high-resolution image from a single low-resolution input (left). Traditional methods tend to produce over-smoothed images that lack high frequency textures and do not look natural (second picture). One focus of our research was the development of algorithms that are able to create realistic textures (third picture) rather than a pixel-accurate reproduction of ground truth (right picture).
The wide dissemination of video cameras and the amount of visusal content on the internet have made digital images and videos the main carriers of information over the last few decades. We are interested in a range of signal and image processing problems both in computational photography and scientific imaging. Our focus is on methods that aim at computationally enhancing the quality of images and recovering probable original images by undoing the adverse effects of image degradation such as blur.
An intriguing problem in image restoration is super-resolution, aiming at recovering a high-resolution image from low-resolution input. Many traditional performance measures such as peak-signal-to-noise ratio (PSNR) correlate poorly with the human perception of image quality. Algorithms minimizing these metrics thus tend to produce over-smoothed images that lack high-frequency textures and do not look natural despite yielding high PSNR values. By focussing on realistic textures rather than pixel-wise accuracy we achieved a significant boost in image quality [ ] (see figure above). Enforcing time consistency led to novel approaches for the problems of video super-resolution [ ] and video prediction [ ].
Another focus area has been the problem of blind deblurring. Removing blur is hard as usually neither the sharp image nor the blur kernel is known. We have developed recurrent network architectures that propagate information between multiple consecutive blurry observations which helps restore the desired sharp image or video [ ]. Additionally we developed a framework to estimate the modulation transfer function (MTF), which plays an important role for lens quality assessment and non-blind deblurring, directly from natural images [ ].
Another line of work is concerned with image processing applied to magnetic resonance images [ ]. We have worked on MRI sequence generation [ ], hardware optimization [ ] and acceleration of acquisition processes [ ].
In [ ] a novel way of MRI image reconstruction using a forward model of the shim coil-modulated imaging process was used. An optimization-based approach was employed in [ ] to improve the quality of MR images by compensating for subject motion-induced artifacts. In [ ] a differentiable digital twin of the entire MRI measurement and reconstruction apparatus was proposed, which allowed for novel ways to design and improve MRI sequences.
In collaboration with Peer Fischer's group at our Stuttgart site, we have recently delved into the fields of acoustic holography and optical computing. Acoustic holography allows for manipulation of acoustic fields in unprecedented detail. Casting the hologram computation for a given target intensity pattern as an inverse problem allows us to leverage optimization and machine learning tools. Our work on optical computing is concerned with the training and physical implementation of neural networks as optical systems. The development of optical non-linearities and suitable architectures plays a key role in increasing the flexibility of such methods.