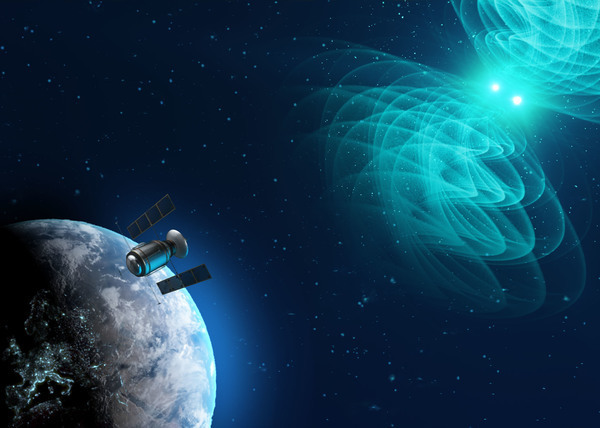
Neural network deciphers gravitational waves from merging neutron stars in a second
Machine learning method could revolutionize multi-messenger astronomy
- 06 March 2025
- Empirical Inference
Binary neutron star mergers emit gravitational waves followed by light. To fully exploit these observations and avoid missing key signals, speed is crucial. In a study to be published in Nature on March 5, 2025, an interdisciplinary team of researchers presents a novel machine learning method that can analyze gravitational waves emitted by neutron star collisions almost instantaneously – even before the merger is fully observed. A neural network processes the data and enables a fast search for visible light and other electromagnetic signals emitted during the collisions. This new method could be instrumental in preparing the field for the next generation of observatories.
Binary neutron star mergers occur millions of light-years away from Earth. Interpreting the gravitational waves they produce presents a major challenge for traditional data-analysis methods. These signals correspond to minutes of data from current detectors and potentially hours to days of data from future observatories. Analyzing such massive data sets is computationally expensive and time-consuming.
An international team of scientists has developed a machine learning algorithm, called DINGO-BNS (Deep INference for Gravitational-wave Observations from Binary Neutron Stars) that saves valuable time in interpreting gravitational waves emitted by binary neutron star mergers. They trained a neural network to fully characterize systems of merging neutron stars in about a second, compared to about an hour for the fastest traditional methods. Their results will be published in Nature on March 5, 2025, under the title “Real-time inference for binary neutron star mergers using machine learning”.
Why is real-time computation important?
Neutron star mergers emit visible light (in the subsequent kilonova explosion) and other electromagnetic radiation in addition to gravitational waves, as shown in this video. “Rapid and accurate analysis of the gravitational-wave data is crucial to localize the source and point telescopes in the right direction as quickly as possible to observe all the accompanying signals,” says the first author of the publication, Maximilian Dax, who was a Ph.D. student in the Empirical Inference Department at the Max Planck Institute for Intelligent Systems (MPI-IS) and who is now a PostDoc at ETH Zurich and the ELLIS Institute Tübingen.
The real-time method could set a new standard for data analysis of neutron star mergers, giving the broader astronomy community more time to point their telescopes toward the merging neutron stars as soon as the large detectors of the LIGO-Virgo-KAGRA (LVK) collaboration identify them.
“Current rapid analysis algorithms used by the LVK make approximations that sacrifice accuracy. Our new study addresses these shortcomings,” says Jonathan Gair, a group leader in the Astrophysical and Cosmological Relativity Department at the Max Planck Institute for Gravitational Physics in the Potsdam Science Park.
Indeed, the machine learning framework fully characterizes the neutron star merger (e.g., its masses, spins, and location) in just one second without making such approximations. This allows, among other things, to quickly determine the sky position 30% more precisely. Because it works so quickly and accurately, the neural network can provide critical information for joint observations of gravitational-wave detectors and other telescopes. It can help to search for the light and other electromagnetic signals produced by the merger and to make the best possible use of the expensive telescope observing time.
Catching a neutron star merger in the act
“Gravitational wave analysis is particularly challenging for binary neutron stars, so for DINGO-BNS, we had to develop various technical innovations. This includes for example a method for event-adaptive data compression,” says Stephen Green, UKRI Future Leaders Fellow at the University of Nottingham. Bernhard Schölkopf, Director of the Empirical Inference Department at MPI-IS and at the ELLIS Institute Tübingen, adds: “Our study showcases the effectiveness of combining modern machine learning methods with physical domain knowledge.”
DINGO-BNS could one day help to observe electromagnetic signals before and at the time of the collision of the two neutron stars. “Such early multi-messenger observations could provide new insights into the merger process and the subsequent kilonova, which are still mysterious,” says Alessandra Buonanno, Director of the Astrophysical and Cosmological Relativity Department at the Max Planck Institute for Gravitational Physics.
Here is the link to the Nature paper.
gravitational waves
DINGO-BNS
Nature
machine learning
neural network
Empirical Inference
LIGO
KAGRA
Max Planck Institute for Gravitational Physics