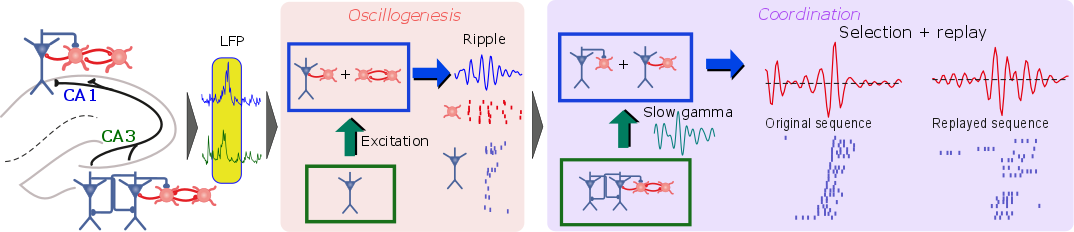
The mechanisms involved in replay of memory traces in the hippocampus. Left: connectivity of excitatory (blue) and inhibitory (red) cells in regions CA3 and CA1. Center: key circuits involved in generating synchronized neuronal activity. Right: circuits involved in generating replays of sequential activity of a sparse population encoding a memory trace. See [ ].
Brain networks are characterized by a dense connectivity that generate complex dynamics and high dimensional data. Machine learning helps us uncovering their organization and function in multiple ways.
The analysis of three-dimensional (3D) electron microscopy data allowed reconstructing with high spatial resolution the morphological features of neurons and their connections [ ]. Such detailed properties need to be incorporated in biologically realistic computational models to uncover brain mechanisms. However, the complexity of such models does not provide direct answers, but instead requires interpretation assisted by sophisticated data analysis techniques. This is well illustrated by our investigation of the replay of memory traces in the hippocampus [ ], in which key neural circuits underlying the replay of memorized events during sleep are uncovered with the help of supervised machine learning.
These computational modelling studies need to be further complemented by extensive analyses of large-scale recordings in order to detect and uncover the role of transient interactions between multiple regions across the whole brain. Our causal inference methods were used in this context to improve our understanding of how memory trace replay participates in brain-wide phenomena that consolidate new memories during sleep [ ].
Another fascinating question of current neuroscience is uncovering the representations encoded by neurons in higher level regions such as the prefrontal cortex (PFC). Machine learning algorithms enabled unprecedented characterizations of these representations during conscious visual perception. This has been done notably through the use of unsupervised learning approaches such as Non-Negative Matrix Factorization [ ] as well as supervised learning techniques that allow assessing the perceptual information content of neural populations [ ].
Finally, high-dimensionality of electrophysiology and neuroimaging data is a major challenge for their analysis. We addressed it with novel theoretical frameworks providing neuroscientists with highly interpretable multivariate analyses techniques. In particular, MultiView independent component analysis [ ] allows for example to incorporate neuroimaging data originating from multiple subjects to fit identifiable probabilistic models of brain response to stimuli. Moreover, we developed a statistical framework based on random matrix theory and point processes to assess the significance of multivariate measures of the coupling of spiking activity recorded simultaneously in large ensembles of neurons and mesoscopic electric field activity [ ]. This tool leverages high-dimensional statistics in order to efficiently compute interpretable analyses of the most recent multi-channel electrophysiological recording technologies [ ].