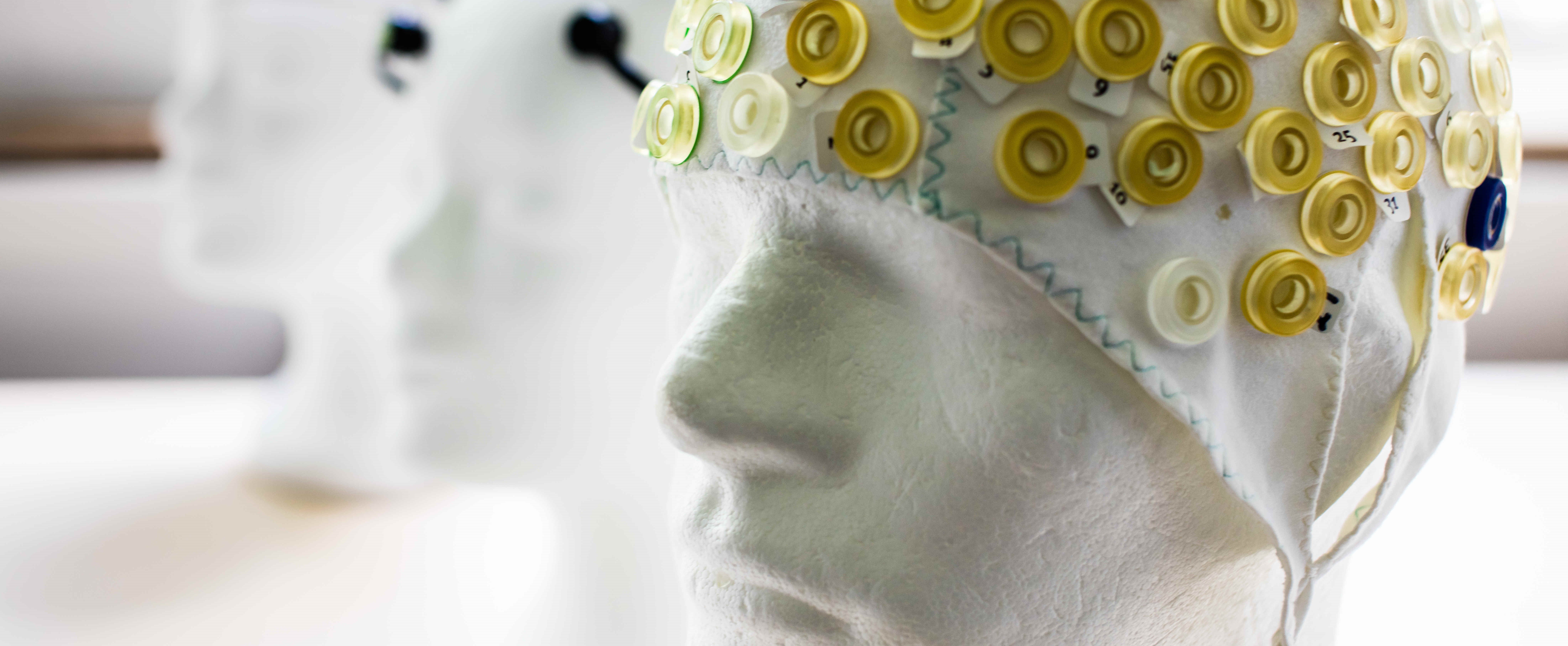
Brain-computer interfaces (BCIs) hold promise in restoring communication for completely locked-in stage (CLIS) patients in late stages of amyotrophic lateral sclerosis (ALS). Despite more than two decades of research, however, late-stage ALS patients remain incapable of operating BCIs, arguably because such systems currently rely on brain processes that are impaired as a result of disease progression. To establish communication with CLIS-ALS patients, it is thus crucial to, first, understand how ALS affects neurophysiological as well as cognitive processes and, second, develop BCI systems that target brain processes which remain functional into late stages of the disease.
In a series of studies, we have investigated how ALS affects neural and cognitive processes. In particular, we were able to show that the neural correlates of self-referential processing are already impaired in the early stages of ALS, i.e., neural deficits emerge before cognitive deficits manifest in overt behavior [ ]. Following up on this work, we recorded electrophysiological signals in two CLIS-ALS patients and demonstrated that the locked-in stage is accompanied by a major slow-down of the brain's dominant rhythm [ ]. The cognitive implications of this slow-down are yet to be understood.
Based on these insights, we developed more accessible BCI control strategies for late-stage ALS patients by targeting brain processes that are likely to remain functional into late disease stages [ ]. We successfully validated this system in a long-term study with two ALS patients, who achieved stable communication for more than one year [ ].
To translate this system from lab to home use, we have pioneered a transfer learning approach for BCIs that drastically reduces the amount of training data while maintaining decoding accuracy [ ] and developed a novel brain-decoding feature, based on task-induced frequency modulations of canonical brain rhythms, that is particularly useful for low-channel setups [ ].
To evaluate the unsupervised use of this system across multiple days, we developed an open-source framework that couples an easy-to-use application with consumer-grade recording hardware [ ]. A large study showed that participants were able to use the system without expert supervision, and results indicated that the framework can enable research on BCI robustness, environmental effects, and behavioral correlates, all of which could hardly be captured in traditional laboratory settings. These advances should enable us to build performant cognitive BCIs with off-the-shelf hardware, eventually leading to BCI systems available for large-scale application outside of laboratory environments.