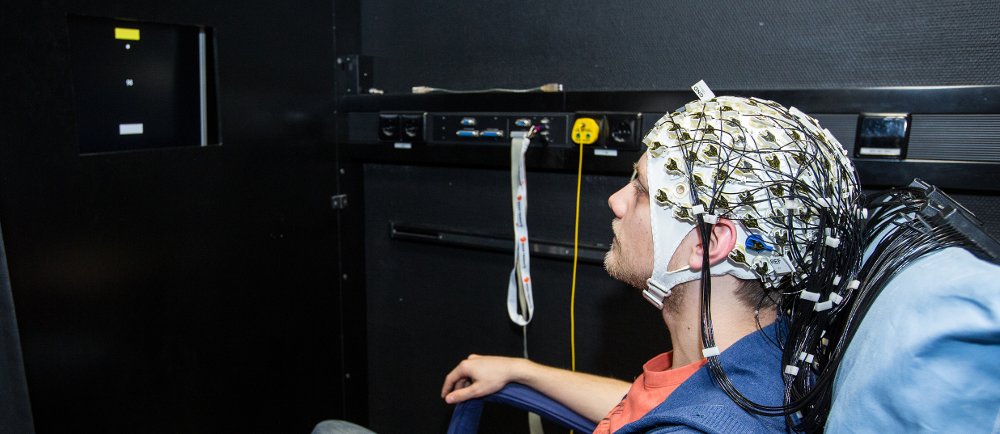
Brain-computer interfaces (BCIs) translate neural recordings into signals that may be used for communication and/or the control of neuroprosthetic devices. Research in this domain poses interesting challenges to machine learning, because data is typically scarce, noisy, and non-stationary [ ]. Furthermore, good decoding algorithms are contingent on domain knowledge that is not readily available and difficult to incorporate into traditional statistical methods. Accordingly, we employ machine-learning methods to study neural processes involved in BCI-control and use these insights to develop novel decoding algorithms and enhance experimental paradigms.
\paragraph{Machine learning algorithms for brain-state decoding}
A crucial aspect of our work is the development of algorithms for real-time brain-state decoding. Building upon our experience in Bayesian inference, we have developed a graphical model decoding framework for ERP-based visual speller systems [ ]. This framework incorporates prior information on letter frequencies into the decoding process, thereby enhancing decoding performance. Furthermore, we were the first to successfully apply the framework of multi-task learning to the domain of BCIs [ ]. As the signal characteristics used by subjects to control a BCI share common aspects, the incorporation of data from previously recorded subjects substantially decreases calibration time and enhances overall decoding performance [ ]. Besides working on methods for real-time decoding, we also develop tools to investigate the neural basis of disorders of cognition. This is essential to understand how the diseased brain differs from the one of healthy subjects, which has implications for the design of BCI systems for patient populations. Building upon the framework of Causal Bayesian Networks, we were the first to provide a comprehensive set of causal interpretation rules for neuroimaging studies [ ]. Extending this line of work, we have developed a causal inference method that is able to detect causal relations between two brain processes, even in the presence of latent confounders [ ].
\paragraph{Brain-computer interfaces for communication}
Building upon our machine-learning methods, we have investigated the neural basis of the ability to operate a BCI in healthy subjects and in patient populations. We could show that the configuration of large-scale cortical networks, as represented in high-frequency gamma-oscillations of the brain's electromagnetic field, influences a subject's ability to communicate with a BCI [ ]. Building upon these insights, we have developed a novel class of BCIs for patients in late stages of amyotrophic lateral sclerosis [ ].
\paragraph{Brain-computer interfaces for rehabilitation}
While BCIs were initially conceived as communication devices for the severely disabled, we have argued that they can also be used for stroke rehabilitation [ ]. By combining a BCI with a seven degrees-of-freedom robotic arm, that serves an exoskeleton, we could show a brain-controlled rehabilitation robot supports patient with chronic stroke in self-regulation of sensorimotor brain rhythms [ ]. The concept of brain-controlled rehabilitation robotics can be extended to systems that monitor patients' learning progress adapt the rehabilitation exercise in real-time [ ].